We’re moving from narratives to visual evidence. Image recognition powered by AI doesn’t just speed up the claims process, it has brought up a new level of clarity, consistency, and trust to the insurance process by letting pictures speak louder than paperwork. As insurers grow more confident in AI’s ability to deliver objective and data-backed assessments, trust in automation is no longer a risk—it’s a strategic advantage
Over the years, there has been a transformational shift in the way claims processing is done. The introduction of AI, especially in uncovering patterns in fragmented data, has helped adjusters make the insurance process much easier and faster.
In particular, the integration of AI-powered image recognition has replaced manual inspections and time-consuming paperwork with digital records and automated image analysis, enabling accurate and efficient claims assessments.
How does it Work?
AI, with the help of computer vision and machine learning algorithms, can process visual data to identify objects and detect damage in photos or videos. For instance, in the case of a car accident, it can assess damage from images submitted by policyholders to estimate repair costs and flag potential fraud.
This is possible by training ML models on vast and diverse datasets to recognize the patterns in the images with various damage types and severities. Image recognition APIs labels the damage (e.g., scratches, dents, broken parts) and classifying the extent of the damage, while deep learning improves accuracy over time by learning from different scenarios. Optical Character Recognition (OCR) extracts text from supporting documents like repair bills and integrates it into the claims workflow, further automating and accelerating the end-to-end process. This combination helps insurers make data driven decisions as they are exposed to diverse scenarios and datasets.
Traditional vs. AI-Driven Claims: A Side-by-Side Shift
Aspect | Traditional Claims Process | AI-Powered Claims Process |
Filing a Claim | Requires paperwork, phone calls, and coordination with agents | Submitted via mobile or web apps using images and documents |
Inspection Method | Manual inspections and in-person visits | Image recognition APIs and ML models analyse uploaded visuals automatically |
Time to Assess Damage | Takes several days or weeks | Assessment completed within hours |
Accuracy & Consistency | Subjective assessments prone to human error and inconsistency across adjusters | AI ensures consistency and data-driven evaluations across all regions |
Fraud Detection | Limited and reactive, depends on adjuster vigilance | AI proactively flags suspicious claims and anomalies |
Adjuster’s Role | Time-consuming routine inspections and cross-verification | Focus shifts to handling complex cases while AI handles routine assessments |
Workflow Efficiency | Slower turnaround times, communication delays, manual bottlenecks | Streamlined, faster, and more accurate workflows with minimal manual intervention |
System Integration | Siloed systems, high dependence on legacy processes | Seamless integration with core insurance platforms and digital ecosystems |
Customer Experience | Frustrating delays and lack of transparency | Transparent, faster, and digital-first experience |
Allstate, a global carrier, have been using image recognition in claims processing through the Allstate mobile app, where policyholders submit photos of damage. The app use AI algorithms to analyse and assess the damage and expediate claims process, enhancing accuracy of the damage assessment.
Another notable example is Aviva, integrating visual AI to help in motor and home repair. Here, the technology helps in guiding and assisting engineers in the remote assessment during the repair. This helps in the consistency of the diagnosis also identifying errors or suspicious activities based on the past data patterns which add time to motor claims.
Operational Benefits for Insurers
With the integration of AI image recognition, the benefits are far reaching and substantial. Claim processing time is significantly reduced from days to hours, to faster payouts and improved customer satisfaction. The convenience that is brought through claims submission via phone and apps makes the claims resolution faster and transparent, helping both the policyholder and insurers.
Automation of such complex process also reduces the need for manual checking and inspections and labour costs associated with it. Along with these, accurate damage detection and assessment improves the overall consistency in claims processing and ultimately paves the way for a more efficient, customer-centric insurance ecosystem.
Challenges and Consideration
As the adoption of AI-driven solutions increases, it comes with a critical challenge. Chief among these is the need to protect the privacy of customer data, the complexity of integrating with legacy systems, and model bias and fairness. To start with, sensitive information like pictures and personal data submitted during the claims process requires robust privacy protocols and data protection. At the same time, models should be trained on diverse datasets to ensure fairness for consistent and non-discriminatory results, which can affect the claim outcomes.
AI models should be run through different scenarios to remain objective and function properly. The complexity of integrating into legacy systems can be slowed down by selecting the right AI architecture and plug-and-play platforms, which traditional systems with rigid, built-in silos can never handle.
Conclusion
Insurers now have access to technology that can be customised to complement their operations and ecosystems without a complete overhaul. This approach can not only make the deployment faster but also reduce operational friction—allowing them to tap into their full potential.
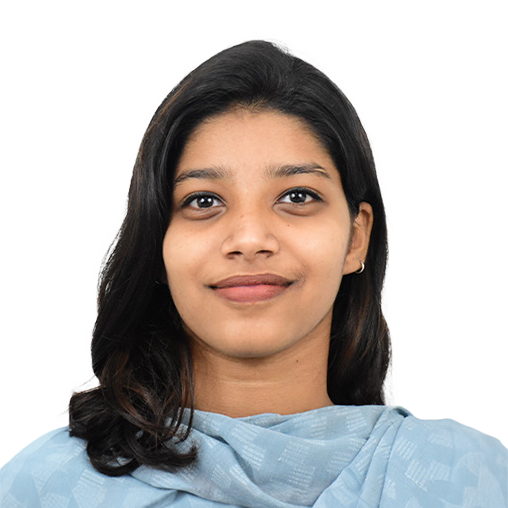
Ardra Girish
Growth Specialist